AI isn’t’ just a buzzword anymore—it’s becoming a core part of how businesses operate and innovate. Whether you’re running a startup or scaling an established brand, building an effective AI business strategy can help you unlock efficiency, gain insights, and deliver more value to your customers.
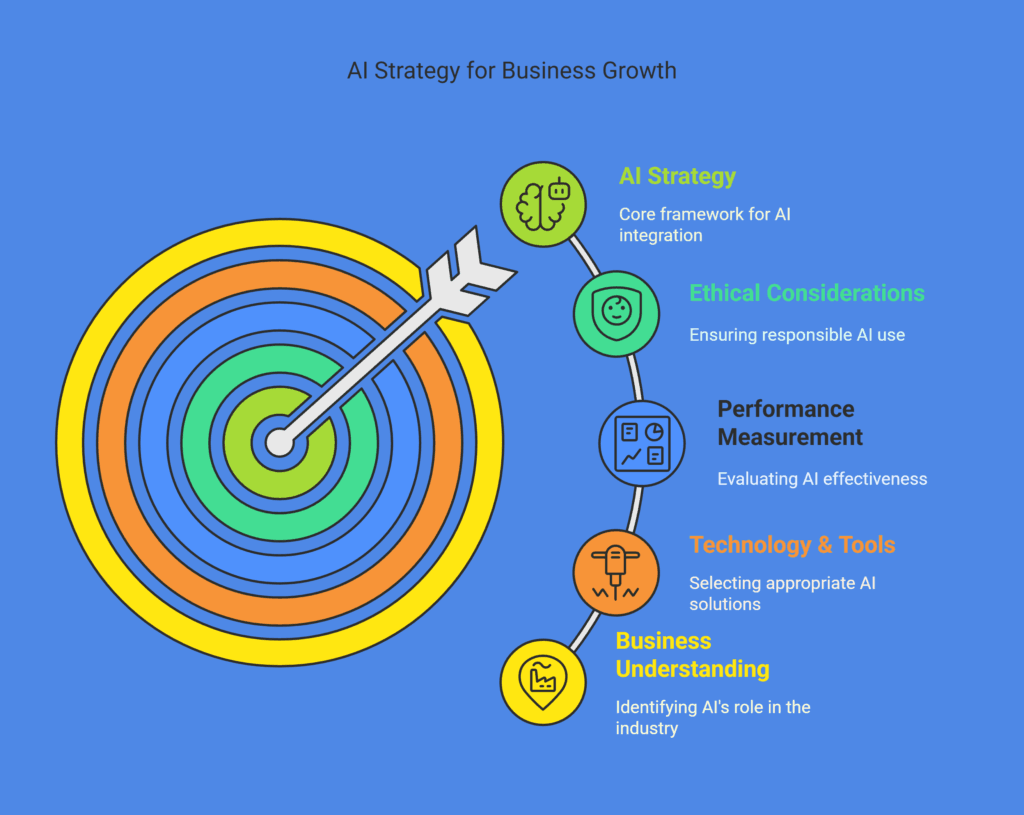
In this blog post, we’ll walk you through a step-by-step guide to creating an AI strategy tailored to your business goals.
1. Understand the Role of AI in Your Industry
Before exploring tools and solutions, take a moment to understand how AI is shaping your industry. Ask yourself:
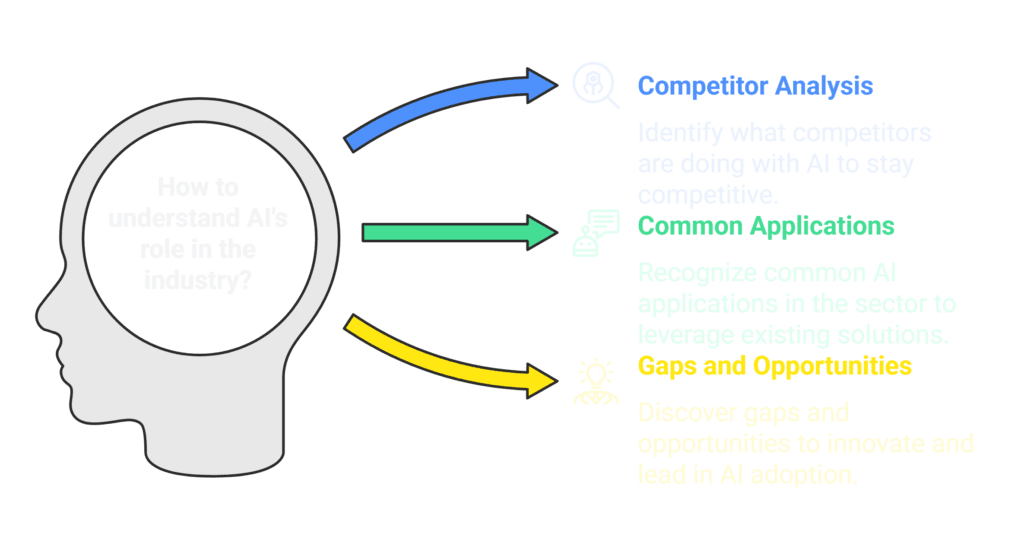
- What are your competitors doing with AI?
- Are there typical AI applications in your sector (like chatbots in customer service, predictive analytics in retail, or computer vision in manufacturing)?
- Where are the gaps and opportunities?
🔍 Tip: Look at case studies from similar businesses. This helps visualise practical applications of AI, not just theoretical ones.
2. Identify Business Challenges That AI Can Solve
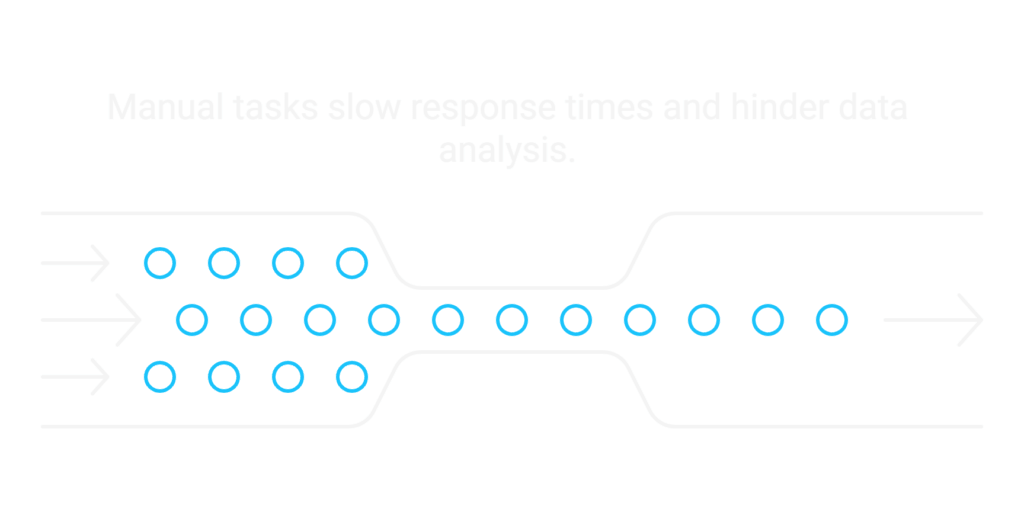
AI is powerful, but it’s not a magic wand. To make the most of it, identify clear pain points in your current operations. Examples include:
- Repetitive manual tasks
- Delayed customer responses
- Data overload with no actionable insights
- Inefficient supply chain or logistics
🎯 Focus on problems where AI can create tangible value, such as cost savings, speed improvements, or better decision-making.
3. Set Clear AI Objectives Aligned with Business Goals
Define what success looks like for your AI initiative. Align AI use cases with your broader business goals:
Business Goal | AI Objective |
---|---|
Improve customer service | Implement AI-powered chatbots |
Boost sales | Use AI for predictive lead scoring |
Streamline operations | Automate repetitive back-office tasks |
Better decision making | Deploy AI for advanced data analytics |
Make sure your goals are SMART (Specific, Measurable, Achievable, Relevant, and Time-bound).
4. Assess Your Data Readiness
AI thrives on data. Without organised and relevant data, your AI efforts will fall flat.
Ask:
- Do we have the necessary data?
- Is it accurate, updated, and accessible?
- Do we need to invest in data cleaning or infrastructure?
🧠 Pro Tip: Start small with existing data. You don’t use massive datasets to begin testing AI solutions.
5. Choose the Right AI Technologies & Tools
You don’t want to build everything from scratch. Depending on your needs and budget, you can:
- Use off-the-shelf tools (like Chatgpt, Google AI, or Azure AI services)
- Collaborate with AI vendors or platforms
- Build custom AI models (if you have the expertise)
🛠️ Tools to Explore:
- For NLP: Openai, Google Cloud NLP
- For analytics: Power BI + AI, Tableau with ML integrations
- For automation: UiPath, Zapier + AI plugins
6. Build a Cross-Functional AI Team
AI projects require collaboration between business leaders, data scientists, developers, and domain experts. Your team should ideally include:
- A project manager (to keep things aligned with business goals)
- Data analyst or data engineer
- AI/ML specialist
- Stakeholders from the problem-area teams (like sales or operations)
👥 Start small, maybe with a task force or pilot team, and grow from there.
7. Start with a Pilot Project
Choose a low-risk, high-impact use case and run a pilot. This helps you test the waters, learn from real-world results, and iterate on your approach.
📊 Example:
Start by automating lead scoring using AI and compare the results with traditional methods over 30 days. Measure conversion rates and time saved.
8. Measure Performance and Iterate
Track your KPIS regularly. Did the AI tool help you save time, reduce errors, or boost revenue? Gather feedback from users and refine the system.
Key metrics might include:
- Time saved
- Accuracy improvement
- Customer satisfaction
- ROI from Aisn’tnestment
🔄 AI isn’t a one-and-done deal. Keep learning, tweaking, and improving.
9. Ensure Ethics, Privacy & Compliance
AI needs to be responsible and transparent. Be mindful of:
- Data privacy laws (like GDPR or local regulations)
- Bias in AI decision-making
- How do you explain AI-driven outcomes to your team or customers
✅ Always have a framework in place for ethical AI use.
10. Scale Gradually
Once your pilot proves successful, scale it to other parts of your business. Use the learnings to fine-tune your broader AI roadmap.
🗺️ Document your AI journey so others in the organisation can replicate the success.
Final Thoughts: AI Strategy is a Business Strategy
Building a business strategy isn’t just about tech—it’s about solving real problems, driving growth, and staying competitive.
You don’t have to be a data scientist to get started. Begin with your business goals, identify where AI can help, and take small, smart steps forward.